Tapping into Insights: Analyzing Unstructured Data
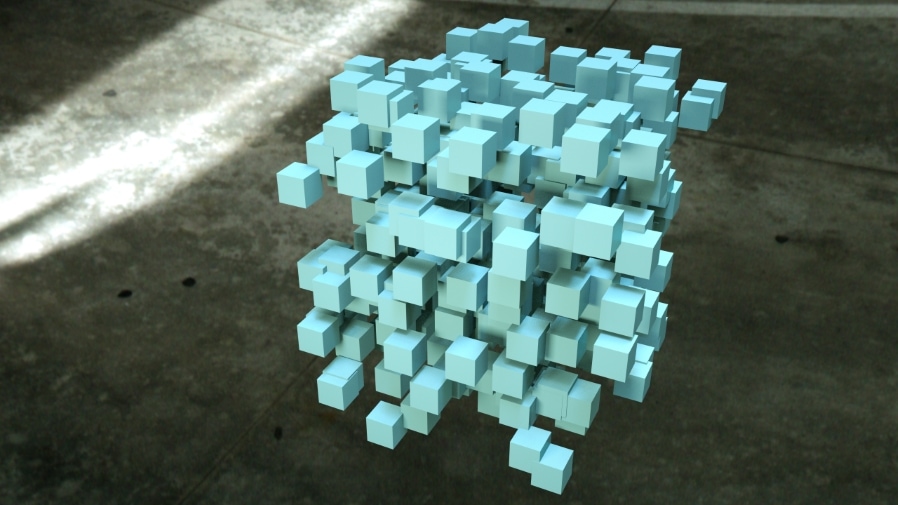
One of the key challenges in analyzing unstructured data is the sheer volume and variety of information.
Unstructured data, such as text, images, and videos, presents a unique set of challenges for analysis. Unlike structured data that is organized in a predefined format, unstructured data lacks a clear structure, making it difficult to extract meaningful insights.
Traditional data analysis methods often struggle to handle the unstructured nature of the data, which can include social media posts, customer reviews, and even audio recordings.
Below, we explore the challenges of analyzing unstructured data, and what technologies and tools are available to analyze such data.
On this page:
The Challenges of Analyzing Unstructured Data
Analyzing unstructured data poses various challenges that hinder effective data interpretation and extraction of valuable insights. Unlike structured data, which is organized and readily accessible, unstructured data refers to information that does not conform to a specific format or schema.
This includes text documents, social media posts, emails, audio recordings, and images. The sheer volume and diversity of unstructured data make it difficult to process and analyze using traditional methods.
Additionally, unstructured data often lacks clear labels or categories, making it challenging to classify and extract meaningful information. This poses a significant hurdle for data analysts who rely on structured data for their analysis.
Furthermore, unstructured data presents challenges in terms of data quality and accuracy. Unlike structured data, which is typically stored in databases and undergoes rigorous validation processes, unstructured data is often sourced from various channels and may contain errors or inconsistencies.
For example, text data extracted from social media platforms may contain abbreviations, slang, and misspellings, making it difficult to extract accurate information. This lack of data quality can lead to inaccurate analysis and unreliable insights.
Moreover, unstructured data often contains noise and irrelevant information, further complicating the analysis process. Data analysts must invest significant time and effort in filtering and cleansing the data to ensure the accuracy and reliability of their analysis.
In conclusion, the challenges posed by unstructured data, such as its volume, lack of structure, and data quality issues, make it a complex task for data analysts to effectively analyze and extract valuable insights.
RELATED: Benefits of Digital Asset Management: 10 Benefits of DAM
Natural Language Processing (NLP) for Text Data Analysis
Natural Language Processing (NLP) techniques offer a powerful approach for extracting meaningful information from textual data.
NLP encompasses a range of methods and algorithms that enable computers to understand, interpret, and generate human language. By applying NLP to text data analysis, researchers and practitioners can gain valuable insights from unstructured data sources such as social media posts, customer reviews, and news articles.
NLP algorithms can automatically process and analyze large volumes of text, enabling organizations to uncover patterns, trends, and sentiments that would be difficult to detect manually. This can be particularly useful for businesses looking to understand customer opinions, identify emerging trends, or monitor brand reputation.
One common application of NLP in text data analysis is sentiment analysis, which involves determining the sentiment or emotion expressed in a piece of text. Sentiment analysis algorithms can classify text as positive, negative, or neutral, allowing organizations to gauge public opinion about their products or services.
Another important NLP technique is named entity recognition, which involves identifying and categorizing named entities such as people, organizations, and locations mentioned in text.
This can be useful for tasks such as extracting information from news articles or identifying key players in a specific industry.
Overall, NLP techniques provide a powerful toolkit for unlocking insights from unstructured textual data, enabling organizations to make data-driven decisions and gain a competitive edge in today’s information-driven world.
Image and Video Analysis for Visual Data
Image and video analysis technologies play a crucial role in extracting relevant information from visual data. These technologies use computer vision techniques to interpret and analyze the content of images and videos, enabling the extraction of valuable insights.
Computer vision, as a subfield of artificial intelligence, focuses on the development of algorithms and models that can understand and interpret visual content, allowing for a deeper analysis of images and videos.
How Image and Video Analysis Technologies Extract Relevant Information
By harnessing the power of cutting-edge image and video analysis technologies, a wealth of pertinent information can be extracted, eliciting a profound sense of awe and wonder in the audience. These advanced technologies have the ability to delve deep into the visual data, uncovering hidden patterns, detecting objects, and even recognizing faces and emotions.
Through sophisticated algorithms and machine learning techniques, images and videos can be analyzed at a scale and speed that surpass human capabilities.
Image analysis technologies can extract relevant information by identifying and categorizing objects within an image. This is achieved through object recognition algorithms that have been trained on vast datasets, enabling them to accurately identify and classify various objects, such as animals, vehicles, or buildings.
Additionally, image analysis can go beyond object recognition and delve into more complex tasks, such as facial recognition and emotion detection. By analyzing facial features and expressions, these technologies can determine the emotions exhibited by individuals in images or videos, providing valuable insights into human behavior and sentiment.
RELATED: PimEyes Facial Recognition AI: What is PimEyes?
Video analysis technologies, on the other hand, can extract relevant information by analyzing the temporal aspect of visual data. They can track objects and individuals across frames, enabling the extraction of trajectory information and the identification of movement patterns.
This is particularly useful in applications such as surveillance or sports analysis, where understanding the dynamics of movement is crucial. Furthermore, video analysis can also involve activity recognition, where algorithms are trained to identify specific actions or behaviors within a sequence of frames.
Overall, the capabilities of image and video analysis technologies are constantly expanding, allowing for the extraction of a multitude of relevant information from visual data. From object recognition to facial emotion detection and activity recognition, these technologies have the potential to provide valuable insights and empower various industries.
By tapping into the power of these cutting-edge technologies, the audience can witness the liberation of information from the visual realm, satisfying their subconscious desire for freedom.
The Role of Computer Vision in Analyzing Visual Content
Computer vision plays a pivotal role in examining and comprehending the visual content, allowing for a comprehensive understanding of the intricate details and patterns present in the images and videos.
By utilizing computer algorithms and deep learning techniques, computer vision systems can extract relevant information from visual data, enabling a wide range of applications such as object recognition, image segmentation, and scene understanding.
These technologies have revolutionized the way we analyze and interpret visual content, providing valuable insights that were previously inaccessible.
One of the key advantages of computer vision in analyzing visual content is its ability to process large amounts of data quickly and accurately. With the advancements in hardware and software technologies, computer vision algorithms can now process images and videos in real-time, enabling applications such as surveillance, autonomous vehicles, and augmented reality.
This speed and accuracy allow for efficient analysis of visual data, leading to improved decision-making and problem-solving capabilities.
Furthermore, computer vision enables a deeper understanding of visual content by uncovering hidden patterns and relationships within images and videos. By analyzing the spatial and temporal properties of visual data, computer vision algorithms can identify objects, detect anomalies, and recognize complex scenes.
This level of analysis goes beyond simple image recognition and allows for a more nuanced understanding of the content, providing valuable insights that can be used in various domains such as healthcare, manufacturing, and entertainment.
Computer vision plays a crucial role in analyzing visual content, providing a comprehensive understanding of the intricate details and patterns present in images and videos. Its ability to process large amounts of data quickly and accurately, along with its capability to uncover hidden patterns and relationships, makes it a powerful tool for gaining valuable insights.
As computer vision technologies continue to advance, we can expect further improvements in analyzing unstructured data, unlocking even more possibilities for understanding and harnessing the power of visual content.
Extracting Valuable Insights from Unstructured Data
Utilizing advanced analytical techniques, valuable insights can be extracted from unstructured data, providing a deeper understanding of complex patterns and trends.
Unstructured data refers to the vast amounts of information that is not organized in a predefined manner, such as text documents, social media posts, emails, and audio recordings.
Traditional data analysis methods are not suitable for handling this type of data, as it lacks a clear structure and is often composed of freeform text or multimedia content. However, with the advent of natural language processing, machine learning, and artificial intelligence, it has become possible to analyze unstructured data and uncover valuable insights.
By tapping into unstructured data, organizations can gain a holistic view of their customers, market trends, and societal sentiment. For example, sentiment analysis can be applied to social media posts or customer reviews to understand how people feel about a particular product or service. This information can then be used to improve marketing strategies, enhance customer satisfaction, and make data-driven decisions.
Similarly, text mining techniques can be used to analyze large volumes of text data, extracting keywords, themes, and sentiment to identify emerging trends or topics of interest. These insights can be invaluable for businesses looking to stay ahead in a competitive market or for researchers studying social phenomena.
The analysis of unstructured data allows for a deeper understanding of complex patterns and trends. By leveraging advanced analytical techniques, organizations can extract valuable insights from unstructured data, enabling them to make more informed decisions and gain a competitive edge.
The ability to tap into unstructured data provides a powerful tool for understanding customer behavior, market trends, and societal sentiment. As organizations strive for freedom and success, the analysis of unstructured data becomes an essential component of their data-driven strategies.
Overcoming Challenges and Limitations
To effectively analyze and derive meaningful information from unstructured data, researchers and organizations must address and overcome various challenges and limitations associated with its nature and format.
Unstructured data lacks a predefined structure and is often in the form of text documents, social media posts, emails, and other textual content. This poses a challenge as it requires advanced natural language processing techniques to extract relevant information and make sense of the data.
Additionally, unstructured data is often vast in volume, making it difficult to analyze and derive insights from manually. The sheer size of the data can overwhelm traditional analytical techniques and tools, necessitating the use of big data analytics platforms and technologies.
Furthermore, unstructured data can be highly heterogeneous, coming from different sources and in various formats. This diversity introduces challenges in data integration and standardization, as different data sources may have different structures and meanings.
To overcome this, researchers and organizations must employ data preprocessing techniques, such as text mining and information extraction, to transform unstructured data into a more structured format suitable for analysis.
Another limitation of unstructured data is the presence of noise and irrelevant information. Textual data often contains noise in the form of typos, grammatical errors, and other inconsistencies. Filtering out this noise and identifying the relevant information is crucial for accurate analysis and insightful findings.
By addressing these challenges and limitations, researchers and organizations can effectively tap into the insights hidden within unstructured data and leverage them for decision-making and innovation.
The Future of Unstructured Data Analysis
Advancements in technology and techniques have opened up new possibilities for analyzing unstructured data.
The use of artificial intelligence, machine learning, and natural language processing has made it easier to extract valuable insights from unstructured data sources such as social media, emails, and documents.
This has led to the growing importance of unstructured data in business decision-making, as organizations recognize the potential for uncovering hidden patterns and trends that can inform strategic planning and improve operational efficiency.
Advancements in Technology and Techniques
The field of data analysis has witnessed significant progress in technology and techniques, leading to enhanced opportunities for extracting valuable insights from unstructured data.
With the advancements in machine learning algorithms and natural language processing techniques, it is now possible to analyze unstructured data sources such as social media posts, customer reviews, and news articles in a more efficient and accurate manner.
These technologies enable data analysts to uncover patterns, trends, and sentiments hidden within vast amounts of unstructured data, providing valuable information that can drive business decisions and strategies.
One of the key advancements in technology is the development of deep learning algorithms, which are capable of automatically extracting features and patterns from unstructured data. These algorithms can analyze text, images, and audio data, allowing for a more comprehensive analysis of unstructured data sources.
Additionally, the availability of large-scale computing resources and cloud-based platforms has made it easier to process and analyze immense amounts of unstructured data in real-time, enabling organizations to gain insights and make data-driven decisions more quickly.
Another significant advancement is the integration of natural language processing techniques into data analysis workflows. Natural language processing algorithms can understand and interpret human language, enabling data analysts to extract meaning and sentiment from unstructured text data.
This allows for a more nuanced analysis of customer feedback, social media conversations, and other textual sources, providing organizations with a deeper understanding of their customers’ opinions, preferences, and behaviors.
The advancements in technology and techniques have opened up new possibilities for analyzing unstructured data. With the power of machine learning algorithms and natural language processing techniques, organizations can tap into the valuable insights hidden within unstructured data sources, driving innovation and strategic decision-making.
By leveraging these advancements, businesses can gain a competitive edge and meet the ever-growing demands of a data-driven world.
The Growing Importance of Unstructured Data in Business Decision-Making
The utilization of unstructured data in business decision-making is becoming increasingly significant as organizations strive to gain a competitive edge in the data-driven world.
Unstructured data refers to information that does not have a predefined data model or organization, such as text documents, emails, social media posts, and multimedia content. Unlike structured data, which is organized in a tabular format, unstructured data is often messy and complex, making it challenging to analyze using traditional data analysis methods.
However, advancements in technology and techniques, such as natural language processing and machine learning algorithms, have made it possible to extract valuable insights from unstructured data.
The growing importance of unstructured data in business decision-making can be attributed to its ability to provide a more comprehensive understanding of customer preferences, market trends, and competitive landscapes. By analyzing unstructured data, organizations can uncover hidden patterns and sentiments that may not be evident through structured data alone.
For example, sentiment analysis of customer reviews can reveal valuable insights about product satisfaction and identify areas for improvement. Social media monitoring can provide real-time feedback on brand perception and help identify emerging trends.
These insights can inform strategic decision-making, product development, marketing campaigns, and customer relationship management, ultimately enhancing the organization’s competitive advantage.
As the volume and variety of unstructured data continue to grow exponentially, businesses that can effectively tap into these insights will be better equipped to make informed decisions and adapt to the ever-changing business landscape.
Conclusion
Analyzing unstructured data poses significant challenges due to its lack of organization and structure. However, the application of natural language processing (NLP) techniques has proven to be effective in extracting valuable insights from textual data. The analysis of visual data, such as images and videos, also presents its own set of challenges. However, advancements in image and video analysis technologies have made it possible to extract meaningful insights from visual data. This has opened up new opportunities for researchers and businesses to gain valuable information from unstructured data sources.
Although there are limitations and challenges associated with analyzing unstructured data, such as the complexity of data organization and the need for specialized tools and techniques, the future of unstructured data analysis appears promising.
As technology continues to advance, it is likely that more sophisticated algorithms and methodologies will be developed, allowing for more accurate and efficient analysis of unstructured data. This will ultimately lead to better decision-making and improved outcomes in various fields, including healthcare, finance, marketing, and social sciences.