Harnessing Unstructured Data: Management Insights
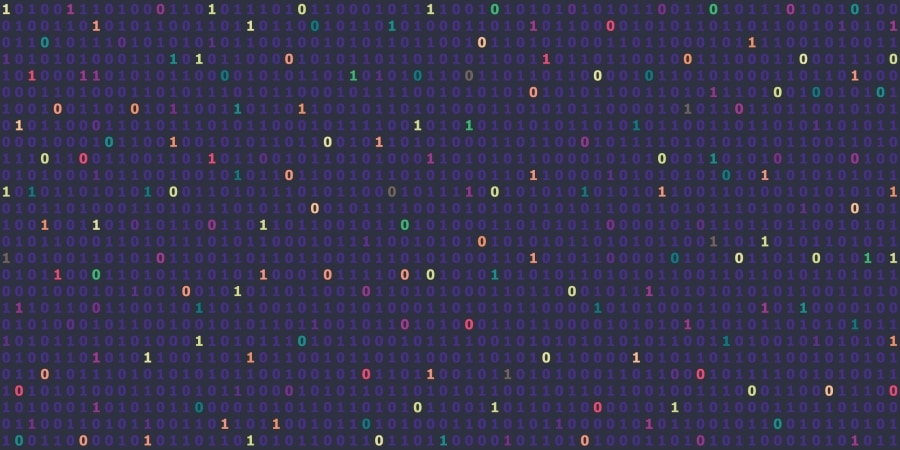
Unstructured data refers to information that does not have a predefined format or organization, making it difficult to analyze and extract insights from. With the exponential growth of data in today’s digital age, the importance of harnessing unstructured data has become increasingly evident. This article aims to provide management insights on the process of harnessing unstructured data, highlighting the tools, technologies, and strategies that can be employed to unlock its value.
In order to fully comprehend the significance of harnessing unstructured data, it is essential to first understand its nature. Unlike structured data, which is organized and stored in a predefined format such as databases or spreadsheets, unstructured data exists in various forms like text documents, social media posts, images, videos, and emails. This abundance of unstructured data holds immense potential for organizations seeking to gain a competitive edge. However, without effective management and analysis, this data remains untapped and its value unrealized.
To address this challenge, various tools and technologies have emerged to assist in the management of unstructured data. These include natural language processing (NLP) algorithms, machine learning techniques, and data visualization tools. By leveraging these tools, organizations can effectively process and analyze unstructured data, transforming it into valuable insights that can drive informed decision-making.
Furthermore, the article will explore different strategies for extracting insights from unstructured data, such as sentiment analysis, topic modeling, and entity recognition. Through the examination of successful case studies and best practices, this article aims to provide management insights on how organizations can effectively harness the power of unstructured data.
On this page:
Understanding Unstructured Data
Understanding unstructured data is crucial for organizations looking to harness the valuable insights hidden within vast amounts of textual information.
Unstructured data refers to any form of information that does not fit into a traditional, structured database. This type of data includes text documents, emails, social media posts, and other textual information that does not have a predefined format or organization.
Unlike structured data that can be easily organized and analyzed using traditional methods, unstructured data poses unique challenges due to its lack of structure and organization.
Organizations that are able to effectively understand and analyze unstructured data can gain valuable insights that can drive decision-making and improve business processes. By harnessing the power of natural language processing and machine learning techniques, organizations can extract meaningful information from unstructured data sources and use it to identify trends, patterns, and sentiments.
This can help organizations understand customer preferences, improve product development, and enhance customer experience. Additionally, understanding unstructured data can also help organizations identify potential risks and opportunities in real-time, allowing them to respond quickly and effectively to changing market conditions.
Understanding unstructured data is crucial for organizations seeking to unlock the hidden insights within vast amounts of textual information. By leveraging advanced technologies and analytical techniques, organizations can effectively extract meaningful information from unstructured data sources and use it to drive informed decision-making.
As organizations continue to generate and collect large volumes of unstructured data, the ability to harness and analyze this data will become increasingly important for achieving a competitive advantage in today’s data-driven business landscape.
Importance of Harnessing Unstructured Data
The importance of harnessing unstructured data lies in the value it provides for business insights.
By analyzing unstructured data, organizations can gain valuable insights into customer behavior, market trends, and other crucial information that can drive strategic decision-making.
Additionally, the benefits of utilizing unstructured data in decision-making processes are numerous, as it allows for a more comprehensive understanding of complex issues, improved accuracy in forecasting, and the ability to uncover hidden patterns and relationships that may not be apparent when only analyzing structured data.
Overall, harnessing unstructured data can greatly enhance business intelligence and inform more informed and effective decision-making.
The Value of Unstructured Data in Business Insights
Harnessing unstructured data allows businesses to uncover valuable insights that can drive strategic decision-making and enhance competitive advantage.
Unlike structured data, which is organized and easily quantifiable, unstructured data refers to information that does not fit into predefined categories or formats. Examples of unstructured data include social media posts, customer reviews, emails, and audio recordings.
While unstructured data may seem chaotic and difficult to analyze, it holds immense value for businesses seeking to gain a deeper understanding of their customers, market trends, and industry dynamics.
The value of unstructured data in business insights lies in its ability to provide a more holistic and nuanced view of the business environment. By analyzing unstructured data, organizations can gain valuable insights into customer sentiment, preferences, and behaviors.
For example, by analyzing social media posts and customer reviews, businesses can uncover patterns and trends that go beyond simple demographic information. This information can be used to identify emerging market trends, improve product development and marketing strategies, and enhance customer experience.
Harnessing unstructured data allows businesses to tap into the collective knowledge and opinions of their customers, providing them with a competitive edge in the market. By leveraging unstructured data, organizations can make data-driven decisions that are based on real-time, up-to-date information, enabling them to adapt quickly to changing market conditions and stay ahead of their competitors.
RELATED: Normalized Data vs Denormalized Data: Choosing the Right Data Model
Benefits of Utilizing Unstructured Data in Decision-Making
Utilizing unstructured data in decision-making enables businesses to unlock a wealth of untapped knowledge, empowering them to make informed choices that can propel their success in an ever-evolving marketplace.
Unlike structured data, which is organized and easily quantifiable, unstructured data refers to information that does not conform to a predefined data model or format. This includes text documents, social media posts, emails, audio recordings, and images.
By harnessing this vast and diverse range of unstructured data, businesses can gain valuable insights that go beyond traditional sources of information, such as surveys and sales data.
The benefits of utilizing unstructured data in decision-making are manifold.
Firstly, unstructured data provides a more comprehensive understanding of consumer sentiment and preferences. By analyzing social media posts, customer reviews, and online forums, businesses can gain real-time insights into how their products or services are perceived in the market. This enables them to identify trends, address customer concerns, and tailor their offerings to meet the ever-changing demands of their target audience.
Secondly, unstructured data can uncover hidden patterns and relationships that may not be immediately apparent in structured data. By employing advanced analytics techniques, such as natural language processing and machine learning, businesses can extract valuable information from unstructured data sources. This can lead to the discovery of new market opportunities, optimization of business processes, and identification of potential risks or threats.
Lastly, harnessing unstructured data allows businesses to stay agile and adapt to changing market conditions. By continuously monitoring and analyzing unstructured data, organizations can identify emerging trends and adjust their strategies accordingly, ensuring they remain competitive in a rapidly evolving marketplace.
The utilization of unstructured data in decision-making offers businesses a valuable tool for gaining a deeper understanding of their customers, uncovering hidden insights, and staying agile in an ever-changing market. By embracing this wealth of unstructured information, organizations can make informed choices that drive their success and give them a competitive edge.
Tools and Technologies for Managing Unstructured Data
Data Mining and Text Analytics are essential in extracting valuable insights from unstructured data by identifying patterns and trends.
Natural Language Processing (NLP) and Machine Learning techniques enable the automated processing and analysis of textual data, allowing for improved understanding and interpretation.
Lastly, Data Visualization and Reporting tools provide a means to visually represent and communicate the findings from unstructured data analysis, facilitating decision-making processes.
Data Mining and Text Analytics
Data mining and text analytics techniques enable researchers to extract valuable insights from large volumes of unstructured data, unveiling hidden patterns and trends that might otherwise go unnoticed. With the exponential growth of data in today’s digital age, traditional methods of data analysis are becoming increasingly inadequate for handling the vast amount of unstructured data.
Data mining techniques involve the use of algorithms and statistical models to discover patterns, relationships, and correlations within the data. This allows researchers to identify trends and make predictions based on the patterns they uncover.
Text analytics, on the other hand, focuses specifically on extracting information from unstructured text data, such as emails, social media posts, and documents. By applying natural language processing and machine learning algorithms, text analytics can analyze text data to identify sentiments, extract key concepts, and categorize information. This enables researchers to gain valuable insights from text data that would otherwise be challenging to analyze manually.
Overall, data mining and text analytics techniques provide researchers with powerful tools for exploring and extracting insights from unstructured data, enabling them to make informed decisions and uncover hidden opportunities.
RELATED: What is Data Mining, and How is it used by Businesses?
Natural Language Processing (NLP) and Machine Learning
Natural Language Processing (NLP) and Machine Learning techniques have revolutionized the analysis of unstructured text, allowing researchers to unlock valuable information and patterns that were previously hidden.
NLP involves the use of algorithms and computational methods to enable computers to understand, interpret, and generate human language. It encompasses a wide range of tasks, including sentiment analysis, named entity recognition, topic modeling, and text classification. These techniques rely on statistical models and machine learning algorithms to process and analyze large volumes of textual data, enabling researchers to gain insights and make data-driven decisions.
Sentiment Analysis: NLP techniques can be used to determine the sentiment or emotion expressed in a piece of text, such as positive, negative, or neutral. This can be useful for businesses to understand customer feedback or for analyzing public sentiment towards a particular topic.
Named Entity Recognition: NLP algorithms can identify and extract named entities, such as names of people, organizations, and locations, from unstructured text. This can be valuable for information extraction and entity linking tasks.
Topic Modeling: By using NLP techniques, researchers can identify the main topics or themes in a collection of documents. This can be helpful for organizing and categorizing large volumes of text, as well as for exploring trends and patterns.
Text Classification: NLP and machine learning can be combined to automatically classify documents into predefined categories. This can be useful for tasks such as spam filtering, sentiment analysis, and content categorization.
By leveraging NLP and machine learning techniques, researchers can effectively process and analyze unstructured text, gaining valuable insights and uncovering hidden patterns. These methods have the potential to revolutionize various industries, including marketing, customer service, healthcare, and finance.
As the technology continues to advance, the possibilities for harnessing unstructured data are limitless, providing organizations with the freedom to make data-driven decisions and drive innovation.
Data Visualization and Reporting
Visualization and reporting techniques provide a visual representation of complex information, allowing researchers to present data in a clear and concise manner, facilitating understanding and aiding in decision-making processes.
Data visualization is the process of creating visual representations of data, such as charts, graphs, and maps, to help communicate patterns, trends, and insights that may not be immediately apparent from raw data. By transforming data into visual forms, researchers can better identify patterns, outliers, and correlations, enabling them to gain a deeper understanding of the data and make more informed decisions.
Data reporting, on the other hand, involves the presentation of data in a structured and organized format, often in the form of reports or dashboards. These reports provide a summary of the key findings and insights from the data analysis process, allowing researchers to communicate their findings effectively to stakeholders and decision-makers. Reporting techniques often involve the use of descriptive statistics, such as averages, percentages, and trends, to provide a comprehensive overview of the data.
Moreover, interactive dashboards allow users to explore the data further by drilling down into specific details or filtering data based on certain criteria. This interactive approach to reporting empowers users to gain a sense of freedom and control over the data, enabling them to extract meaningful insights and make data-driven decisions.
RELATED: Data Visualization Basics
Strategies for Extracting Insights from Unstructured Data
This discussion will focus on strategies for extracting insights from unstructured data, specifically in the areas of data cleaning and preprocessing, sentiment analysis and opinion mining, and topic modeling and trend analysis.
Data cleaning and preprocessing involve techniques to remove noise and inconsistencies from unstructured data, ensuring its quality and accuracy.
Sentiment analysis and opinion mining techniques aim to extract subjective information from text, such as emotions or opinions, for further analysis.
Lastly, topic modeling and trend analysis techniques enable the identification of key themes and patterns within unstructured data, providing valuable insights for decision-making processes.
Data Cleaning and Preprocessing
Data cleaning and preprocessing is a crucial step in harnessing unstructured data for management insights. It involves transforming raw, unstructured data into a clean, structured format that can be easily analyzed. This process ensures that the data is accurate, consistent, and complete, which is essential for making informed business decisions.
To achieve effective data cleaning and preprocessing, there are several key steps that need to be followed. Firstly, data needs to be cleaned by removing any irrelevant or duplicate information. This helps to reduce noise and improve the quality of the dataset.
Secondly, data needs to be standardized and normalized, ensuring that it is in a consistent format. This step helps to eliminate inconsistencies and discrepancies that may arise from different data sources.
Additionally, data cleaning and preprocessing involve handling missing values by either imputing them or removing them from the dataset. This helps to avoid bias and ensures that the analysis is based on complete and reliable data.
Finally, data needs to be transformed and aggregated to create meaningful variables that can be used for analysis. This involves applying mathematical operations and statistical techniques to derive new insights from the data.
Overall, data cleaning and preprocessing play a vital role in converting unstructured data into valuable information that can drive strategic decision-making in organizations.
Data cleaning and preprocessing are essential steps in harnessing unstructured data for management insights. By removing irrelevant information, standardizing data, handling missing values, and transforming variables, organizations can ensure that their analysis is based on accurate and reliable information.
Through effective data cleaning and preprocessing, businesses can gain valuable insights and make informed decisions that can drive their success. Therefore, it is crucial for organizations to invest time and resources in these processes to unlock the full potential of unstructured data.
Sentiment Analysis and Opinion Mining
Moving from the process of data cleaning and preprocessing, we now delve into the intriguing field of sentiment analysis and opinion mining.
Sentiment analysis refers to the computational study of subjective information present in text data, aiming to identify and extract the sentiments, opinions, and emotions expressed by individuals. As unstructured data continues to grow exponentially, sentiment analysis plays a crucial role in harnessing the power of this information.
By analyzing sentiments, organizations can gain valuable insights into customer opinions, market trends, and public perception, enabling them to make informed decisions and improve their products, services, and overall customer experience.
Opinion mining, also known as sentiment mining, is closely related to sentiment analysis but focuses on extracting and understanding people’s opinions, evaluations, and appraisals rather than just emotions. It goes beyond sentiment classification to provide a deeper understanding of the reasons behind the expressed opinions.
This field involves the use of natural language processing techniques, machine learning algorithms, and computational linguistics to identify and extract opinion-related information from unstructured text data. Opinion mining can be applied in various domains, such as product reviews, social media posts, customer feedback, and online forums, enabling organizations to gain insights into customer preferences, satisfaction levels, and areas for improvement.
Overall, sentiment analysis and opinion mining are powerful tools in harnessing the vast amount of unstructured data available today, providing organizations with valuable insights to drive business decisions and enhance customer experiences.
Topic Modeling and Trend Analysis
Topic modeling and trend analysis are crucial techniques in the field of text analysis, allowing researchers to uncover hidden themes and patterns within large collections of textual data.
Topic modeling is a statistical method that automatically identifies topics or themes present in a set of documents. It helps researchers understand the main ideas and concepts discussed across various documents, even when those documents are unstructured and lack predefined categories. By applying topic modeling, researchers can gain insights into the underlying structure of textual data and identify key topics that emerge from the analysis.
Trend analysis, on the other hand, focuses on identifying patterns and changes in data over time. In the context of text analysis, trend analysis helps researchers understand the evolution of topics and themes in a collection of documents. It enables them to identify emerging trends, popular topics, and shifts in public opinion or interest.
By analyzing trends, researchers can make predictions, identify potential opportunities or risks, and gain a deeper understanding of the dynamics within a given field. Overall, topic modeling and trend analysis are powerful tools that enable researchers to extract meaningful insights from large volumes of unstructured textual data, helping them uncover hidden patterns and trends that may otherwise go unnoticed.
RELATED: Building an Effective Enterprise Data Model
Successful Applications of Unstructured Data Analysis
These case studies demonstrate how organizations have effectively utilized unstructured data analysis to gain valuable insights in these areas, leading to better decision-making and improved business outcomes.
Social Media Listening and Brand Reputation Management
Social media listening and brand reputation management have become essential components of modern business strategies, as organizations aim to harness unstructured data from various online platforms to gain valuable management insights. By monitoring social media platforms such as Facebook, Twitter, and Instagram, businesses can gather real-time information about customer sentiments, preferences, and opinions. This allows companies to stay updated on the latest trends and adapt their strategies accordingly, ensuring that they remain relevant and competitive in the market.
Benefits of social media listening and brand reputation management includes:
- Improved customer satisfaction: By actively monitoring social media conversations, organizations can quickly identify and address customer complaints or issues. This proactive approach demonstrates a commitment to customer service and can help businesses retain their customers and enhance their overall satisfaction.
Competitive advantage: Social media listening provides organizations with valuable insights into their competitors’ strategies, customer feedback, and market trends. By analyzing this data, businesses can identify gaps in the market and develop innovative solutions to meet customer needs, giving them a competitive edge. - Crisis management: Social media platforms can amplify negative feedback or complaints, potentially damaging a brand’s reputation. By monitoring social media conversations, organizations can quickly identify and address any potential crisis situations, mitigating the damage and protecting their brand image.
- Influencer marketing: Social media listening allows organizations to identify influential individuals or groups who can help promote their brand. By engaging with these influencers, businesses can expand their reach and tap into new customer segments, increasing brand visibility and awareness.
Social media listening and brand reputation management play a crucial role in harnessing unstructured data for management insights. By staying connected with their customers and monitoring online conversations, organizations can gain valuable information that can drive strategic decision-making, improve customer satisfaction, and maintain a competitive advantage in the market.
Customer Feedback Analysis and Product Development
Customer feedback analysis is a vital tool for organizations looking to improve their products and meet customer needs more effectively. By analyzing customer feedback, organizations gain valuable insights into the strengths and weaknesses of their products, allowing them to make informed decisions about product development.
Customer feedback analysis provides a way for organizations to understand how their products are perceived in the market and identify areas for improvement. This analysis can help organizations identify trends, patterns, and common customer complaints, enabling them to address these issues and enhance the overall customer experience.
Moreover, customer feedback analysis plays a crucial role in product development. By listening to customer feedback, organizations can gather ideas and suggestions for new features or improvements to existing products. This direct input from customers allows organizations to align their product development efforts with customer preferences, ensuring that the final product meets their needs and expectations.
Additionally, customer feedback analysis can help organizations prioritize product development initiatives by identifying the most pressing customer concerns. This enables organizations to allocate resources effectively and focus on areas that will have the greatest impact on customer satisfaction.
Overall, customer feedback analysis is a powerful tool that enables organizations to harness the voice of the customer, driving product innovation and improving customer satisfaction.
Risk Management and Fraud Detection
In the realm of harnessing unstructured data, the analysis of customer feedback and its integration into product development strategies has been a paramount focus. However, it is crucial to expand our understanding beyond this domain and explore the potential applications of unstructured data in risk management and fraud detection.
Unstructured data refers to the vast amount of information that is not organized in a predefined manner, such as emails, social media posts, or online reviews. By effectively harnessing this data, organizations can gain valuable insights into identifying and mitigating risks, as well as detecting fraudulent activities.
In risk management, the analysis of unstructured data can provide organizations with a comprehensive understanding of potential risks that may arise. By mining data from various sources, such as news articles, industry reports, or even internal documents, organizations can identify emerging risks and take proactive measures to address them.
Furthermore, the analysis of unstructured data can help organizations in assessing the impact of risks and developing appropriate risk management strategies. By analyzing patterns, trends, and sentiments expressed in unstructured data, organizations can enhance their ability to predict and manage risks effectively.
Similarly, in the realm of fraud detection, the analysis of unstructured data can be a powerful tool. Fraudulent activities often leave traces in unstructured data, such as suspicious emails, online transactions, or social media conversations. By harnessing advanced analytics techniques, organizations can uncover hidden patterns, anomalies, and correlations within unstructured data that may indicate fraudulent behavior.
This can enable organizations to detect and prevent fraud in a timely manner, thereby safeguarding their financial resources and reputation.
While the analysis of customer feedback for product development has been a prominent focus in harnessing unstructured data, there is significant potential in applying this approach to risk management and fraud detection. By leveraging the vast amount of unstructured data available, organizations can gain valuable insights that can help them identify and mitigate risks, as well as detect and prevent fraudulent activities.
The ability to harness unstructured data effectively can provide organizations with a competitive advantage in managing risks and ensuring the integrity of their operations.
Best Practices for Harnessing Unstructured Data
To effectively leverage unstructured data, it is crucial to establish and adhere to best practices that enable organizations to derive valuable management insights from this vast and diverse information resource. Unstructured data, such as text documents, emails, social media posts, and audio recordings, poses unique challenges due to its lack of predefined structure and organization. However, by following best practices, organizations can unlock the potential of unstructured data and gain a competitive edge in the market.
- Develop a clear objective: Before diving into analyzing unstructured data, organizations should define their objective and what they hope to achieve through data analysis. Having a clear objective helps in focusing efforts and ensures that the analysis is aligned with the organization’s goals.
- Choose the right tools and technologies: With the advancements in technology, there are now numerous tools and technologies available for analyzing unstructured data. It is essential to select the right tools that suit the specific needs of the organization. Whether it is natural language processing algorithms or machine learning techniques, using the right tools can significantly enhance the accuracy and efficiency of data analysis.
- Implement data preprocessing techniques: Unstructured data often contains noise, inconsistencies, and irrelevant information. To ensure accurate analysis, organizations should implement data preprocessing techniques to clean and transform the data. This may involve removing duplicate records, standardizing formats, and eliminating irrelevant information. Proper preprocessing enhances the quality of the data and improves the reliability of the insights derived from it.
- Continuously evaluate and update processes: Harnessing unstructured data is an ongoing process, and organizations should continuously evaluate and update their processes to stay relevant and adapt to changing business needs. Regularly reviewing the effectiveness of data analysis techniques, exploring new technologies, and incorporating feedback from stakeholders can help organizations stay ahead of the curve and maximize the value derived from unstructured data.
By following these best practices, organizations can effectively harness unstructured data and gain valuable management insights. The ability to analyze and derive meaning from unstructured data is becoming increasingly important in today’s data-driven business landscape.
By leveraging best practices and embracing the power of unstructured data, organizations can gain a competitive advantage and drive innovation in their respective industries.
Conclusion
Harnessing unstructured data is essential for organizations to gain valuable insights and stay competitive in this data-driven era. Understanding the nature of unstructured data and its importance in decision-making processes is crucial.
By utilizing advanced tools and technologies, organizations can effectively manage and analyze unstructured data, unlocking its full potential. Strategies for extracting insights from unstructured data, such as natural language processing and machine learning, enable organizations to uncover valuable information and make informed business decisions.
By adopting these best practices, organizations can fully leverage the power of unstructured data and gain a competitive advantage in today’s data-driven world.