Designing Business Intelligence Architecture
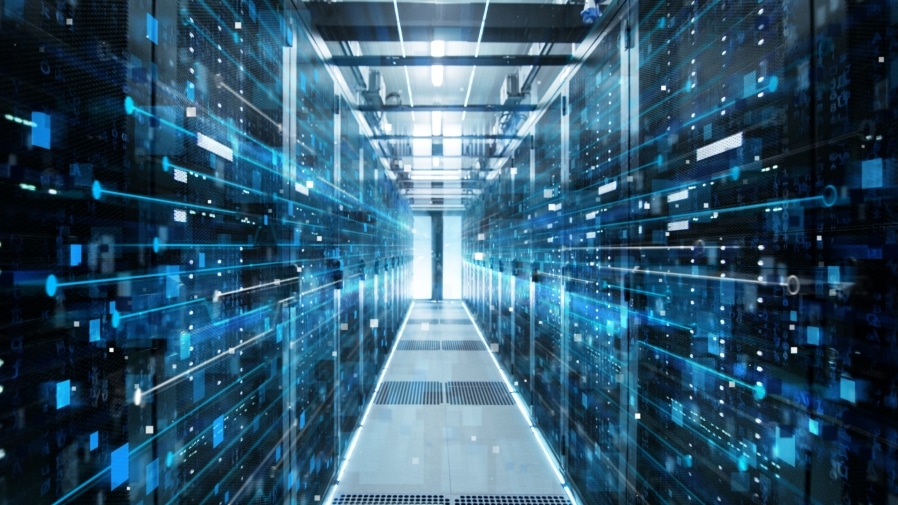
Business Intelligence (BI) has become an integral part of modern businesses, enabling them to make informed decisions based on data-driven insights. Designing an effective BI architecture is crucial for organizations to extract valuable information from vast amounts of data and optimize their operations.
Effective BI architecture not only enhances decision-making but also empowers organizations to serve their customers better by delivering products and services that align with their needs and preferences.
This article explores the key steps involved in designing a robust BI architecture, focusing on identifying business objectives and requirements, assessing data sources and integration, designing the data warehouse, implementing ETL processes, and selecting and configuring reporting tools.
On this page:
Identify Business Objectives and Requirements
The identification of business objectives and requirements is crucial for the successful design of a business intelligence architecture, as it lays the foundation for enabling data-driven decision-making and fostering organizational growth.
By clearly defining the objectives of the business, such as increasing sales, improving customer satisfaction, or reducing operational costs, organizations can align their data collection and analysis efforts accordingly. This ensures that the right data is collected and analyzed to provide valuable insights that support strategic and tactical decision-making processes.
Furthermore, understanding the specific requirements of the business is essential for designing an effective business intelligence architecture. This involves identifying the types of data that need to be collected, the sources from which the data will be obtained, and the frequency at which it needs to be updated. It also includes determining the level of granularity required for data analysis, as well as any specific data transformation or integration needs.
By thoroughly understanding the business requirements, organizations can design a business intelligence architecture that not only meets the current needs but also has the flexibility to adapt to future changes and growth.
Overall, the identification of business objectives and requirements forms the cornerstone of a successful business intelligence architecture, enabling organizations to leverage the power of data for informed decision-making and sustainable growth.
Assess Data Sources and Integration
Assessing data sources and integration is crucial in establishing a robust foundation for informed decision-making, invoking a sense of confidence and reliability in the audience.
By thoroughly evaluating the available data sources, organizations can ensure that they have access to accurate, relevant, and up-to-date information. This assessment involves identifying the various data sources within the organization, such as databases, data warehouses, and external data providers, and evaluating their quality, reliability, and accessibility.
Integration plays a vital role in business intelligence architecture as it involves combining data from multiple sources into a unified and consistent format. This integration process enables organizations to gain a holistic view of their data, identifying relationships and patterns that would otherwise be overlooked.
It also facilitates the seamless flow of information across different systems and departments, ensuring that decision-makers have access to all the relevant data they need. By assessing data sources and integration, organizations can create a comprehensive and reliable foundation for their business intelligence initiatives, ultimately enabling them to make informed decisions and drive business success.
Design the Data Warehouse
To create a robust foundation for informed decision-making and instill confidence in the audience, it is imperative to strategically craft the data warehouse, allowing for seamless integration and a comprehensive view of the organization’s data.
The design of the data warehouse plays a crucial role in the success of a business intelligence architecture. A well-designed data warehouse ensures that data from various sources can be effectively consolidated and analyzed, providing a holistic view of the organization’s operations and performance.
A key aspect of designing a data warehouse is ensuring the integration of data from different sources. This involves identifying and assessing the data sources, understanding their structures and formats, and determining how they can be harmonized and transformed to fit the data warehouse schema. Integration is essential to avoid data silos and inconsistencies, enabling the organization to have a unified and accurate view of its data.
Additionally, the design of the data warehouse should consider the scalability and flexibility required to accommodate future data sources and changes in business requirements. This involves careful planning of the data warehouse architecture, including the selection of appropriate technologies and tools that can handle large volumes of data and support complex analytical processes.
By strategically designing the data warehouse, organizations can ensure that their business intelligence initiatives are supported by a robust and reliable foundation, enabling them to make informed decisions and serve their stakeholders effectively.
Implement ETL Processes
Implementing ETL processes is a crucial step in establishing a reliable and efficient data pipeline for the consolidation and transformation of diverse data sources within a data warehouse.
ETL, which stands for Extract, Transform, and Load, involves extracting data from various sources, transforming it into a format suitable for analysis, and loading it into the data warehouse. This process ensures that the data is standardized, cleansed, and integrated, enabling organizations to gain valuable insights and make informed decisions.
By implementing ETL processes, organizations can achieve several benefits. Firstly, it allows for the consolidation of data from multiple sources, such as databases, spreadsheets, and cloud applications, into a single repository. This centralization of data enables easy access and analysis, reducing the time and effort required to gather information from different sources manually.
Secondly, ETL processes facilitate data transformation, which involves converting data into a consistent format and structure. This transformation step ensures that the data is accurate, complete, and consistent, enhancing its reliability and usability.
Lastly, loading the transformed data into the data warehouse enables efficient storage and retrieval, enabling users to query and analyze the data effectively.
Overall, implementing ETL processes is essential for designing a robust business intelligence architecture. It enables organizations to establish a reliable data pipeline, consolidate diverse data sources, and transform the data into a consistent format. By doing so, organizations can unlock the full potential of their data, gain valuable insights, and make data-driven decisions to better serve their customers and stakeholders.
Select and Configure Reporting Tools
Selecting and configuring reporting tools is a crucial step in establishing a comprehensive data analysis framework, enabling organizations to effectively visualize and communicate insights derived from their data. Reporting tools play a vital role in transforming raw data into meaningful information that can be easily understood and interpreted by stakeholders.
These tools provide a variety of features and functionalities that help in creating visually appealing and interactive reports, dashboards, and visualizations.
When organizations select reporting tools, they need to consider their specific requirements, such as the type and complexity of data they deal with, the level of interactivity needed, and the audience they are targeting. There are various reporting tools available in the market, ranging from simple spreadsheet-based tools to advanced business intelligence platforms.
Organizations should choose a tool that aligns with their data analysis goals and provides the necessary functionalities to meet their reporting needs.
Once the reporting tool is selected, it needs to be configured to ensure optimal performance and usability. Configuration involves setting up data connections, defining data sources, and customizing the reporting interface according to the organization’s branding and design guidelines.
It is important to configure the tool in a way that allows easy access to relevant data and facilitates efficient reporting and analysis.
Selecting and configuring reporting tools is a critical step in designing a business intelligence architecture. These tools enable organizations to transform raw data into meaningful insights that can be effectively communicated to stakeholders.
By carefully considering their requirements and configuring the selected tool appropriately, organizations can establish a robust reporting framework that serves their data analysis goals and helps them make informed decisions.
Frequently Asked Questions
How can we ensure the security and privacy of the data in the business intelligence architecture?
Ensuring the security and privacy of data in business intelligence architecture involves implementing robust access controls, encryption techniques, and regular audits. This safeguards sensitive information and mitigates the risk of unauthorized access or data breaches.
What are some best practices for data governance and data quality in the business intelligence architecture?
Best practices for data governance in business intelligence architecture include establishing data ownership, implementing data quality controls, conducting regular data audits, and ensuring compliance with data protection regulations. These measures help maintain data accuracy, reliability, and security.
How can we effectively handle real-time data and ensure it is integrated into the business intelligence architecture?
Effectively handling real-time data and integrating it into the business intelligence architecture requires implementing technologies like data streaming and event processing. This ensures timely data updates, enables real-time analytics, and improves decision-making capabilities for organizations.
What are the key considerations for scalability and performance in the design of the data warehouse?
Key considerations for scalability and performance in designing a data warehouse include data modeling, indexing strategies, partitioning, hardware and software selection, query optimization, and data compression techniques. These factors ensure efficient data storage, retrieval, and analysis.
What are some common challenges faced during the implementation of ETL processes, and how can they be overcome?
Common challenges in ETL implementation include data quality issues, complex transformations, and performance bottlenecks. These can be overcome by establishing data quality controls, optimizing transformation logic, and utilizing parallel processing techniques to improve performance